Group Member: Mu Cai, Yunyu(Bella) Bai, Xuechun Yang
Source code and dataset available at: https://github.com/mu-cai/cs766_21spring
Related Work
The Traditional DL to Train a Model: ERM
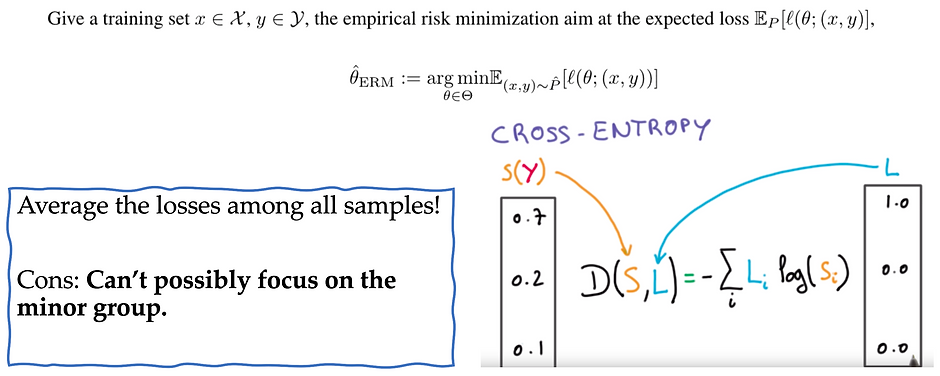
State-of-the-art Methods that Reduce Group Shifts: DRO
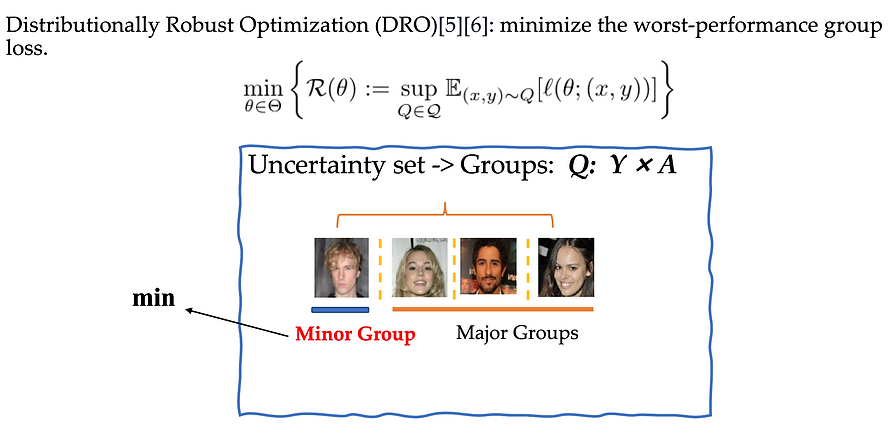
The Performance of the Above Two Methods
Even though DRO focus on minimizing the worst-group loss, the test accuracy of the minor groups is still far from that of the major group!

Current Limitation 1: Synthesized Dataset
The current community utilizes fake data to construct the group-shifted dataset, which doesn’t reflect the distribution of natural images, blocking its real-world applications.
As shown in the picture below, this dataset is constructed by simply stitching a background image and a foreground object.
To facilitate the research in group shifts for the community, we collect a large-scale natural image dataset via web-crawler.

Current Limitation 2: OOD Dataset
Besides, the current research community also doesn’t consider its robustness towards out-of-distribution samples. Real world test images has a wide span of distribution. Therefore, determining whether test images belong to the in-distribution set is critical, which is not yet studied in the community. Here we study the robustness of the neural network models under four diverse high resolution out-of-distribution datasets.
