top of page
Group Member: Mu Cai, Yunyu(Bella) Bai, Xuechun Yang
Source code and dataset available at: https://github.com/mu-cai/cs766_21spring
Our Approach
Strong Regularization
Employ strong L2 penalty during the training process, i.e., large λ value.
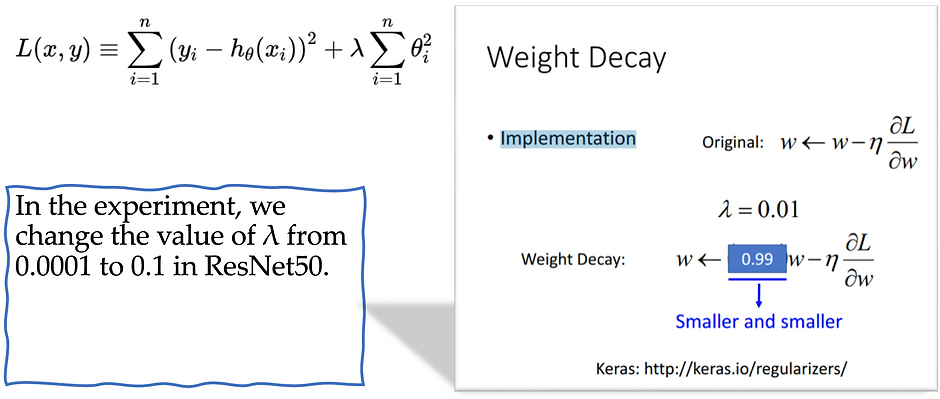
Early Stopping
Stop the training process early enough to prevent overfitting.
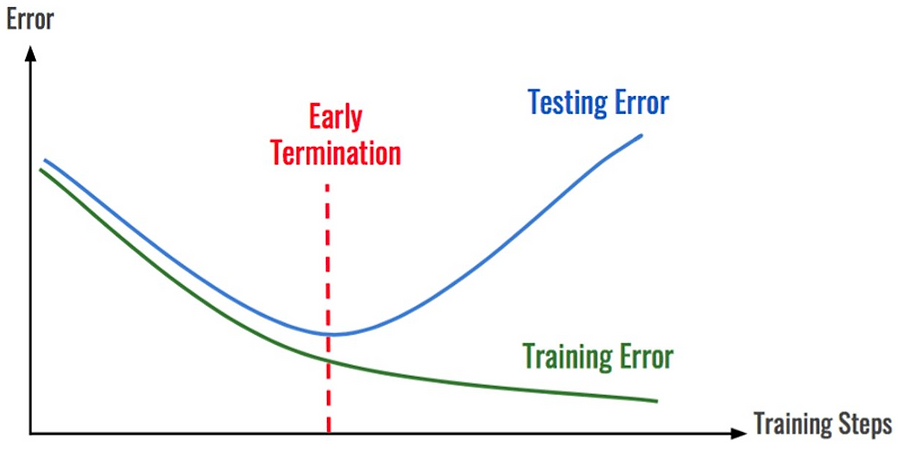
OOD Detection Score
We adopted the MSP score and the EBM score.
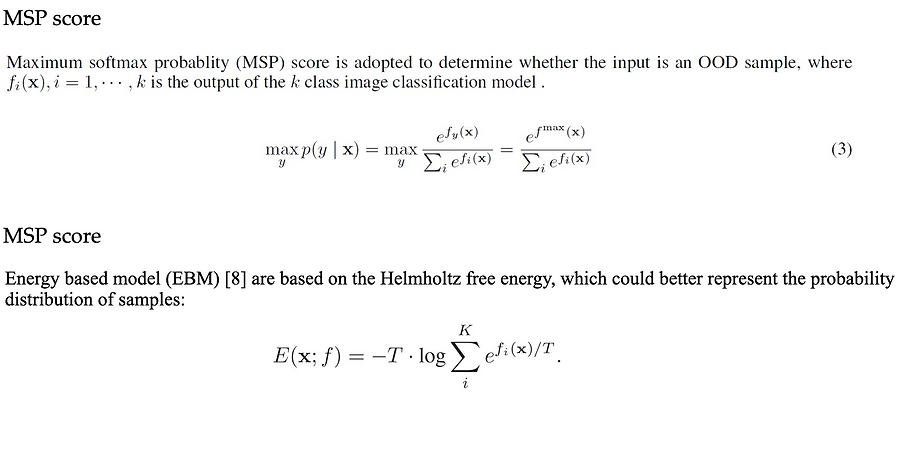
OOD robustness Metrics
We get the ROC (Receiver operating characteristic) curve based on the MSP scores of ID and OOD samples, then compute the AUROC (Area Under the Receiver Operating Characteristics) value.
Besides, we also use FPR95 and AUPRÂ as the OOD robustness metrics.

Our Approach: Careers
bottom of page