Group Member: Mu Cai, Yunyu(Bella) Bai, Xuechun Yang
Source code and dataset available at: https://github.com/mu-cai/cs766_21spring
Strong regularization vs. Early Stopping
As shown in the table below, strong regularization methods boost the test accuracy of the minor groups a lot! The test accuracy on minor groups improved from below 50 percent to over 75 percent for Celebrity Faces Dataset. The test accuracy also increased by about 10 percent for Both models on the Birds data set. While the early stopping also slightly improved the test accuracy, the effects were not significant compared to strong regularization.
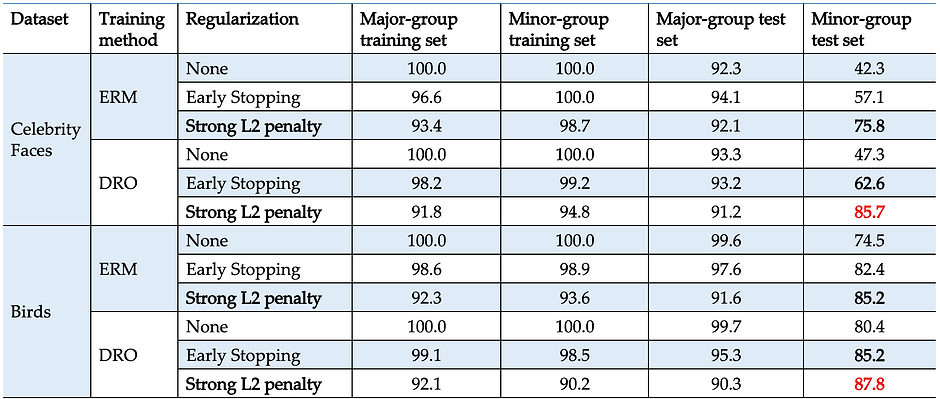
OOD Robustness: MSP vs. EBM
Below are the OOD detection results using the MSP score (left) and the EBM score (right) under the DRO model in Celebrity Faces dataset. The upward arrow means that higher values denote better performance, vice versa. Let's focus on AUROC, whose range is 0-100. We can see that the AUROC values are relatively very low. meaning that The group shifts dataset made the neural network models fragile to OOD samples!
Apparently, the EBM score does a better job than the MSP score.
More quantitive results can found using our GitHub repo at https://github.com/mu-cai/cs766_21spring
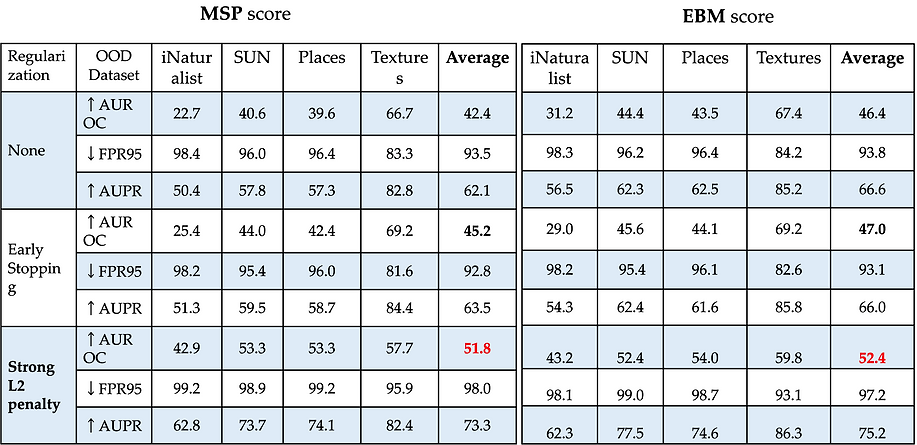
Conclusion
Strong regularization achieves high accuracy for the minor group!​
Neural network models under the group shifts dataset are still fragile under the out-of-distribution dataset.​
Future Work
How to improve the robustness of NN models even without knowing the attributes beforehand?​
How to combine data augmentations to further improve the group robustness?​
Conduct experiment under the dataset with a large number of semantic categories.​
​
Code and dataset are available at https://github.com/mu-cai/cs766_21spring​